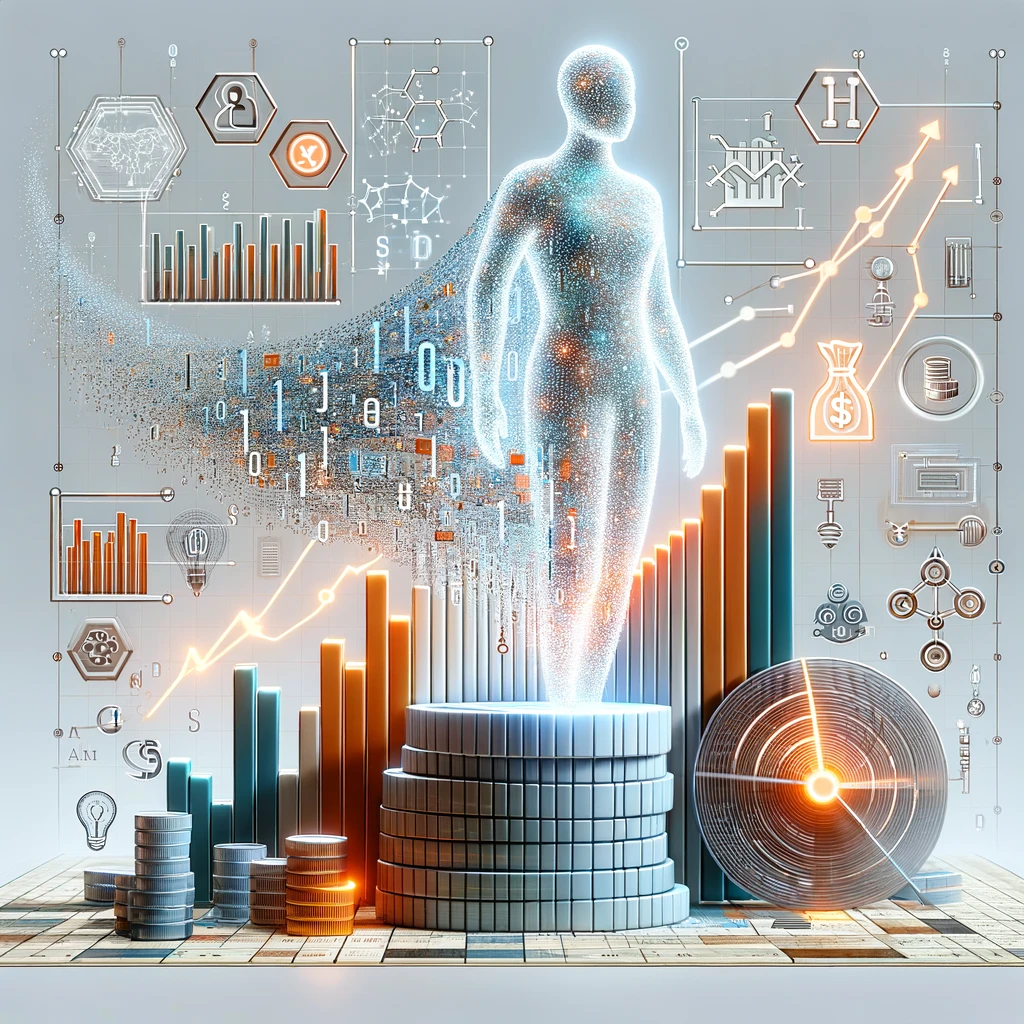
The buzz around Generative AI (GenAI) is growing, and its relevance to our day-to-day jobs in the finance sector is becoming more apparent. This article explores the significant impacts of GenAI across various financial activities, alongside addressing potential weaknesses of this innovative approach.
Portfolio Management: Advisory and Discretionary Management.
Transforming Complex Controls with GenAI.
Weaknesses of GenAI: Access to Data.
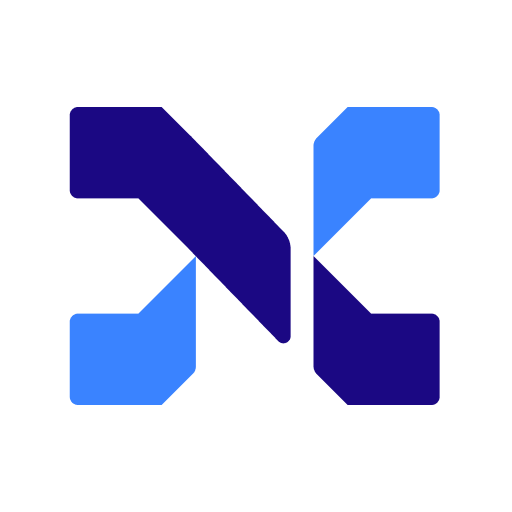
Compliance Activities.
Traditional methods are falling behind in the race against sophisticated money laundering schemes, causing compliance costs to skyrocket. GenAI promises a revolution by analyzing patterns in customer behavior to pinpoint anomalies indicating fraudulent activities. For example, HSBC has implemented AI to sift through millions of transactions, identifying suspicious patterns undetectable by humans. With the right data, GenAI could significantly enhance our ability to detect fraud and complex money laundering activities.
Portfolio Management: Advisory and Discretionary Management.
GenAI is poised to transform portfolio management by issuing trading recommendations, a task currently regulated by legal authorities. It can facilitate the creation of highly personalized investment portfolios by analyzing extensive financial data and predicting market trends. JPMorgan Chase, for instance, uses machine learning to dissect trading patterns and offer tailored trading advice, markedly improving client services and investment results.
Access Review.
The manual verification of appropriate user access across financial systems is both time-consuming and error-prone. GenAI can streamline this process by automating the review of user actions against their access rights, highlighting any discrepancies.
Client Onboarding.
The onboarding process, particularly the scanning and storing of client data, is notoriously time-intensive. Banks are now experimenting with AI technologies that automatically extract information from identification documents to populate client databases, significantly speeding up the process. GenAI could take this a step further by identifying document types without human intervention, simplifying data retrieval. For example, during client onboarding, if a client mentions possessing a green card and working in a public function, GenAI could automatically incorporate PEP and FATCA information into the document and client profile.
GenAi will also ease the storage by adding relevant research criteria automatically.
Transforming Complex Controls with GenAI.
In the financial sector, a significant number of controls are manually executed by users, a process that is not only time-consuming but also prone to errors. Imagine the efficiency gains if these controls could be automated using Generative AI (GenAI). For instance, GenAI can be programmed to verify that income and expense figures align with historical data, ensuring no unexpected variances. It can also ensure that all transactions processed during the day have accurate data, from amounts to account numbers.
Furthermore, GenAI can take on any proportionality control, comparing different sets of data to identify discrepancies or anomalies. Whether it’s ensuring compliance with financial regulations or conducting routine audits, GenAI’s ability to analyze vast datasets in real time can significantly reduce the manual burden on financial professionals. This shift not only accelerates the control process but also enhances accuracy, allowing financial institutions to identify and address issues more swiftly and effectively.
Weaknesses of GenAI: Access to Data.
GenAI’s functionality requires access to extensive datasets, from client names to their transaction histories. This is manageable within a private network or a bank’s cloud infrastructure, but externalizing this function poses a risk of sensitive data exposure to competitors. Consequently, GenAI systems are best deployed on bank premises, though this comes with its own set of cost challenges.
Weaknesses of GenAI: Accountability.
Determining responsibility in the event of an error presents a major concern. If GenAI errs, is the IT department accountable, or does the business unit bear some responsibility for lack of oversight? This conundrum highlights a broader issue of accountability in an era dominated by advanced IT solutions. Organizations must establish clear ownership of GenAI processes, ensuring they are not solely managed by IT departments with limited capacity. Incorporating manual validation into each step of the process might be necessary to mitigate accountability and legal concerns.
Weaknesses of GenAI: Data Storage Challenges.
One of the foundational challenges with integrating GenAI in financial operations is ensuring that data is properly stored and managed within SQL databases. SQL databases, while robust and widely used for managing structured data, present several issues that could hinder the effectiveness of GenAI:
- Data Quality and Consistency: For GenAI to function optimally, it requires high-quality, consistent data. However, SQL databases may contain outdated, duplicate, or inconsistent data due to legacy systems and manual entry errors. Ensuring data integrity and cleaning becomes a critical, yet resource-intensive, task.
- Schema Rigidity: SQL databases are schema-based, meaning they require a predefined structure. This rigidity can limit the flexibility needed by GenAI systems to adapt to new data types or unstructured data, which are common in the dynamic financial sector. Adapting the database schema to accommodate new requirements can be cumbersome and time-consuming.
- Scalability and Performance: The vast amounts of data needed for GenAI analysis can strain SQL databases, especially those not designed for high-volume, high-velocity data. This can lead to performance bottlenecks, slowing down data retrieval and processing, and ultimately affecting the responsiveness of GenAI applications.